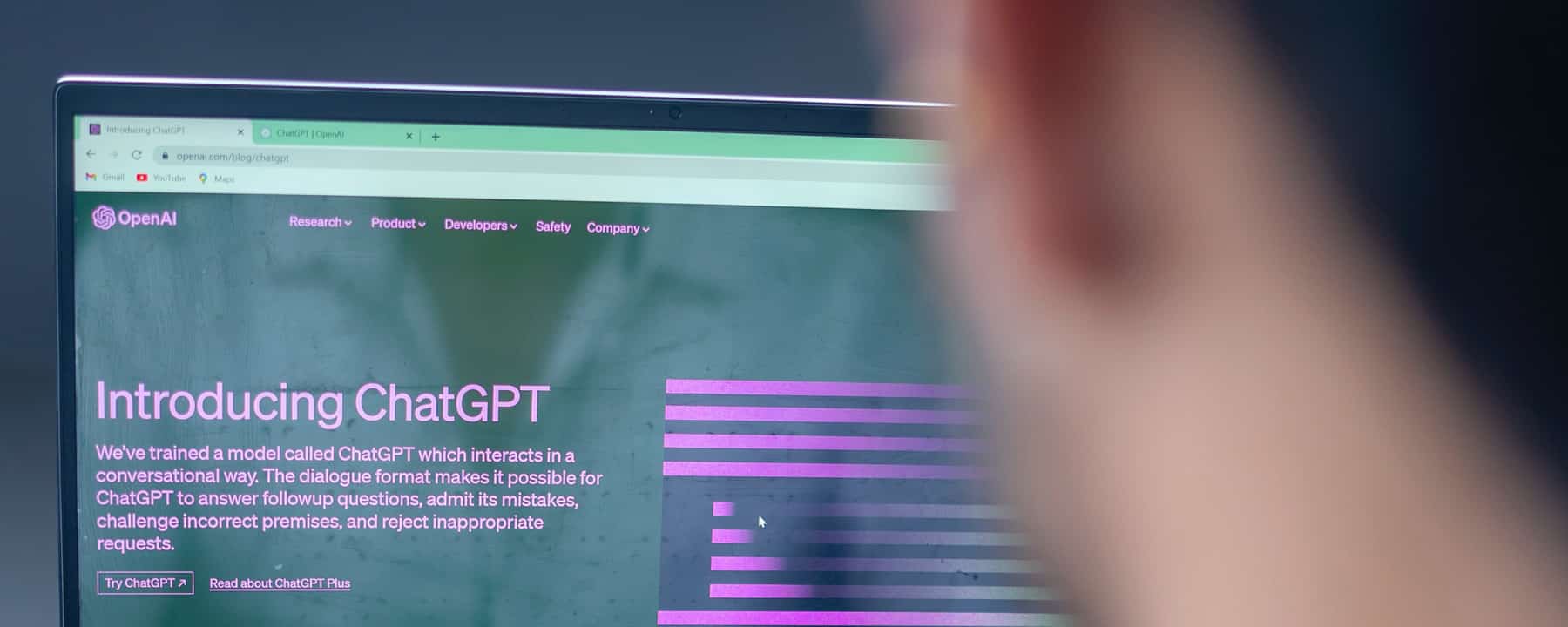
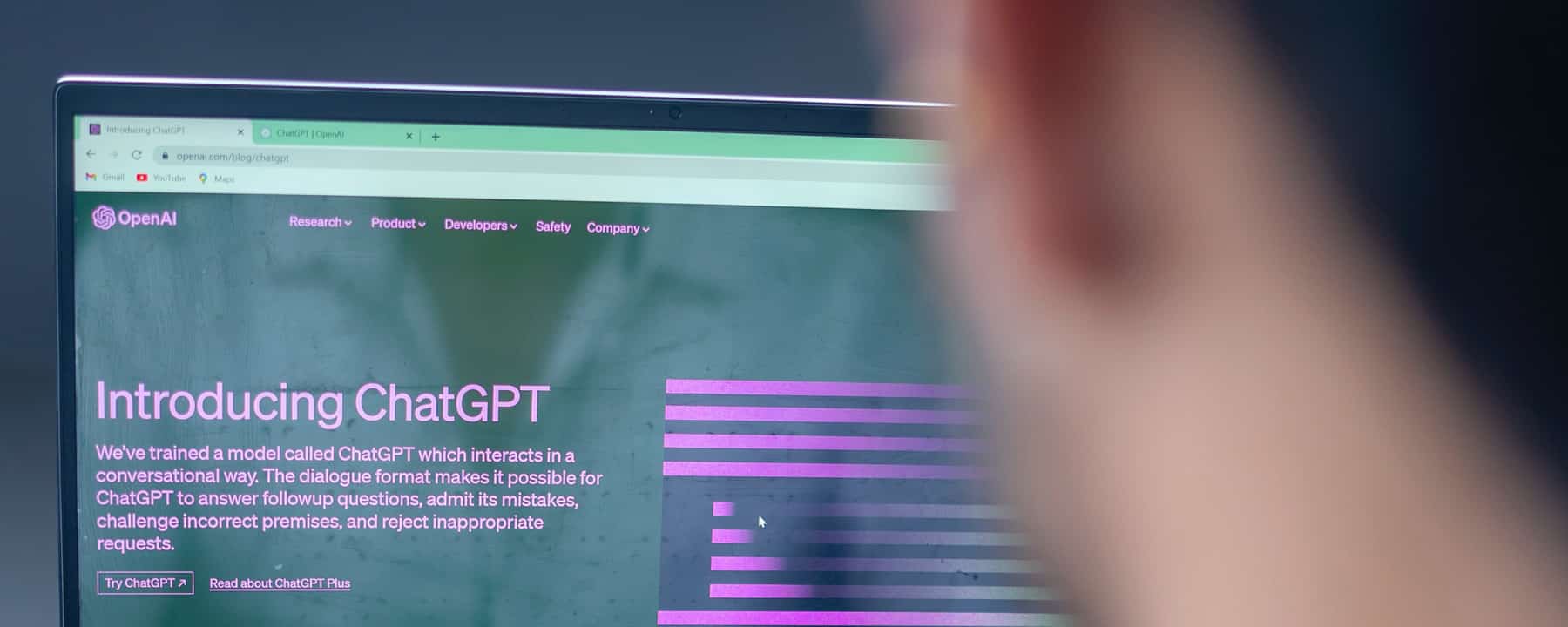
Practical test: how ChatGPT can help with media management
Table of contents
In the digital age, efficient media management plays an important role for companies. The technical term for the professional management of media files is digital asset management (DAM). With good reason, media content is understood here as an asset value. Digital asset management encompasses all the processes required to optimally store, organize, find and distribute digital media such as photos or videos.
To what extent can the use of an AI-based chatbot be helpful in the DAM sector? We will get to the bottom of this question in the following sections using practical examples. In this we focus on the star among chatbots: ChatGPT by OpenAI.*
* BTW: OpenAI is also known for the AI image generator DALL-E.
How can AI help optimize DAM workflows?
Especially in industries that are open to new technologies, artificial intelligence (AI) has been helping to increase the efficiency of DAM workflows for quite some time. This is done, for example, by automatically detecting faces and other objects on digital images (see image recognition). ChatGPT can now be used to speed up other processes. Due to the nature of ChatGPT, these all have something to do with language processing.
Metadata optimization with ChatGPT
As an AI language model, ChatGPT was trained using huge amounts of text, amounting to approximately 500 billion words. This vast textual knowledge can be tapped to develop an ideal metadata schema: one that is unambiguous and contains the categories that are critical to the media assets at hand.
Intermediate note: additional metadata is essential for the efficient organization of digital media. Thus, the search and file management can be significantly improved.
Practical test 1: Creation of a metadata schema with the help of ChatGPT
We did the field test and let ChatGPT (version 4) develop a metadata schema for a specific media archive. The input at the ChatGPT prompt was:
Develop a detailed metadata schema for a commercial digital media archive that has it’s contextual focus on sports photography, especially on Olympic sports.
The scheme that ChatGPT proposed (including explanations) contains 18 categories and looks like this:
- Image identifier: unique ID for each photograph.
- Title: title of the photo. If not provided manually, it can be generated from other metadata elements (like Event, Sport, Athlete(s), and Date).
- Image description: a brief description of the moment captured in the photo.
- Keywords: relevant terms that not covered in other fields that would assist in search and retrieval.
- Date: day when the photograph was taken.
- Location: the geographical location where the event was held.
- Author: photographer who took the photo.
- Source: where the photograph originated from (e.g., from a specific stock photo agency or publication).
- Rights: copyright information, including possible restrictions on use.
- Format: file type and size of the image.
- Resolution: pixel dimensions of the image.
- Color model: specification whether the image is in color or grayscale.
- Event: sport event where the photo was taken. This may include Olympic Games (year and city), World Championships, etc.
- Sport: specific sport captured in the photograph.
- Athlete(s): names of the athletes featured in the photograph.
- Nation: country represented by the athletes.
- Medal Winner: indication if the photo includes any medal winner(s) from the event, and if so, what medal(s) they won.
- Medal Ceremony: Indicaton if the photograph is from a medal ceremony.
Evaluation
While categories 1 to 12 are more or less industry standard and are used in every professional media database, categories 13 to 18 are specifically tailored to the defined use case (stock photo agency / media archive for sports photography).
The schema may be expandable, but it is certainly a solid basis to start from. In this respect, it should be noted that ChatGPT is a useful tool here: the most relevant metadata fields and their respective properties were identified. Thanks to ChatGPT, companies that are new to digital asset management do not have to start from scratch.
Takeaway
With the help of ChatGPT, companies can easily create a metadata schema that fits their specific needs.
Practical test 2: Creation of a keyword tree with the help of ChatGPT
Controlled vocabulary is very important for keywording and metadata optimization. Especially with heterogeneous collections, a well-structured keyword tree (incl. synonym index) is essential. The more diverse the media stock is, the more comprehensive the vocabulary for content indexing will be. The keyword tree is therefore always multi-dimensional in heterogeneous stocks.
We did the second field test and instructed ChatGPT to develop a keyword tree for the said media archive focusing on Olympic sports. Here you can see the result:
1. Sport Discipline
Athletics
-
- Track events
- Field events
- Combined events
Gymnastics
-
- Artistic gymnastics
- Rhythmic gymnastics
- Trampoline gymnastics
Aquatics
-
- Swimming
- Open water swimming
- Synchronized swimming
- High diving
- Water polo
… (and so on for all sports disciplines)
2. Sport Event
Olympic Games
-
- Summer Olympics
- Winter Olympics
World Championships
Continental Championships
National Championships
… (other events)
3. Location
Countries
-
- USA
- Japan
- France
- … (and so on for all countries)
Cities
-
- Tokyo
- Paris
- Los Angeles
- … (and so on for all host cities)
4. Athletes
List of athlete names (controlled vocabulary here would require regular updates)
5. Moment
Action Shot
Victory Moment
Medal Ceremony
Crowd Reaction
Opening Ceremony
Closing Ceremony
… (other significant moments)
6. Outcome
Gold Medal
Silver Medal
Bronze Medal
World Record
National Record
Personal Best
Evaluation
Due to the thematic narrowness, the structure has a rather low depth (only 3 levels) and is divided into 6 main categories. Of course, this schema is not perfect either, but the categories are reasonably chosen and can serve as a starting point for a comprehensive and well-structured keyword tree.
Takeaway
ChatGPT also proves to be a helpful tool when it comes to creating controlled vocabulary – in our case in the form of a keyword tree tailored to the specific needs of a sports photo agency.
Future fields of application of ChatGPT in the DAM sector
In the future, ChatGPT can certainly be integrated in such a way that requests for specific media content are processed automatically. After all, ChatGPT can not only generate linguistically high-quality responses, but could, for instance, also be used to:
-
- initiate a targeted media search
- compile a media selection
- send updates on media content
Always provided that the media stock has textual metadata. Image recognition is namely not ChatGPT’s thing.
In addition, ChatGPT could help making existing knowledge bases more accessible to users, for example, by automatically answering technical questions or providing guidance on media management or use.
Conclusion
The use of ChatGPT can be very helpful, especially when building a media database. As we have seen, ChatGPT can be used to create a content-specific metadata schema that is suitable as a starting point for professional digital asset management. In addition, ChatGPT can jump-start the process of creating a meaningful structure for controlled vocabulary. Other areas of application still need to be tested, but it is already clear that manual tasks that classically fall into the area of media documentation can be partially automated. This saves time and inevitably improves the ROI of marketing assets like photos and videos. It is therefore foreseeable that AI-driven chatbots such as ChatGPT will soon play a decisive role in the DAM sector as well.
Benefit from AI-driven media management today
teamnext’s DAM platform already works with powerful AI modules that speed up work with digital media enormously. The teamnext | Media Hub features automatic face recognition that can be trained on a specific person with just two photos. In addition, our solution has object detection that enables automatic tagging, as well as intelligent image similarity search.
If we’ve made you curious and you’d just like to try out the various AI functions, you can get started immediately with a free 14-day trial for the teamnext | Media Hub with integrated AI platform. In addition, you can of course book an appointment for a free online product demo with one of our experts at any time. Simply use our contact form for this purpose.